My dear friend and colleague Steve Irvine and I will represent our company integrate.ai at the ElevateToronto Festival this Wednesday (come say hi!). The organizers of a panel I’m on asked us to prepare comments about what makes an “AI-First Organization.”
There are many bad answers to this question. It’s not helpful for business leaders to know that AI systems can just-about reliably execute perception tasks like recognizing a puppy or kitty in a picture. Executives think that’s cute, but can’t for the life of them see how that would impact their business. Seeing these parallels requires synthetic thinking and expertise in AI, the ability to see how the properties of a business’ data set are structurally similar to those of the pixels in an image, which would merit the application of similar mathematical model to solve two problems that instantiate themselves quite differently in particular contexts. Most often, therefore, being exposed to fun breakthroughs leads to frustration. Research stays divorced from commercial application.
Another bad answer is mindlessly mobilize hype to convince businesses they should all be AI First. That’s silly.
On the one hand, as Bradford Cross convincingly argues, having “AI deliver core value” is a pillar of a great vertical AI startup. Here, AI is not an afterthought added like a domain suffix to secure funding from trendy VCs, but rather a necessary and sufficient condition of solving an end user problem. Often, this core competency is enhanced by other statistical features. For example, while the core capability of satellite analysis tools like Orbital Insight or food recognition tools like Bitesnap is image recognition*, the real value to customers arises with additional statistical insights across an image set (Has the number of cars in this Walmart parking lot increased year over year? To feel great on my new keto diet, what should I eat for dinner if I’ve already had two sausages for breakfast?).
On the other hand, most enterprises have been in business for a long time and have developed the Clayton Christensen armature of instilled practices and processes that make it too hard to flip a switch to just become AI First. (As Gottfried Leibniz said centuries before Darwin, natura non saltum facit - nature does not make jumps). One false assumption about enterprise AI is that large companies have lots of data and therefore offer ripe environments for AI applications. Most have lots of data indeed, but have not historically collected, stored, or processed their data with an eye towards AI. That creates a very different data environment than those found at Google or Facebook, requiring tedious work to lay the foundations to get started. The most important thing enterprises need to keep in mind is to never to let perfection be the enemy of the good, knowing that no company has perfect data. Succeeding with AI takes a guerrilla mindset, a willingness to make do with close enough and the knack of breaking down the ideal application into little proofs of concepts that can set the ball rolling down the path towards a future goal.
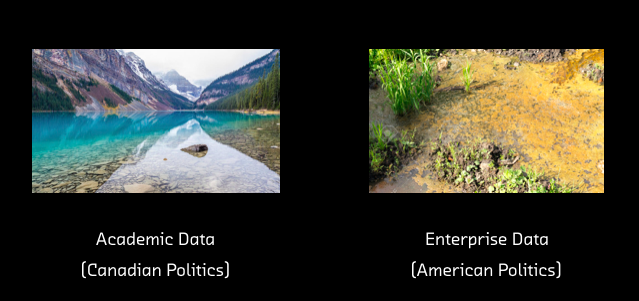
What large enterprises do have is history. They’ve been in business for a while. They’ve gotten really good at doing something, it’s just not always something a large market still wants or needs. And while it’s popular for executives to say that they are “a technology company that just so happen to be financial services/healthcare/auditing/insurance company,” I’m not sure this attitude delivers the best results for AI. Instead, I think it’s more useful for each enterprise to own up to its identity as a Something-Else-First company, but to add a shift in perspective to go from a Just-Plain-Old-Something-Else-First Company to a Something-Else-First-With-An-AI-Twist company.
The shift in perspective relates to how an organization embodies its expertise and harnesses traces of past work.** AI enables a company to take stock of the past judgments, work product, and actions of employees - a vast archive of years of expertise in being Something-Else-First - and either concatenate together these past actions to automate or inform a present action.
To be pithy, AI makes it easier for us to stand on the shoulder of giants.
An anecdote helps illustrate what this change in perspective might look like in practice. A good friend did his law degree ten years ago at Columbia. One final exam exercise was to read up on a case and write how a hypothetical judge would opine. Having procrastinated until the last minute, my friend didn’t have time to read and digest all the materials. What he did have was a study guide comprising answers former Columbia law students had given to the same exam question for the past 20 years. And this gave him a brilliant idea. As students all have to have high LSAT scores and transcripts to get into Columbia Law, he thought, we can assume that all past students have more or less the same capability of answering the question. So wouldn’t he do a better job predicting a judge’s opinion by finding the average answer from hundreds of similarly-qualified students rather than just reporting his own opinion? So as opposed to reading the primary materials, he shifted and did a statistical analysis of secondary materials, an analysis of the judgments that others in his position had given for a given task. When he handed in his assignment, the professor remarked on the brilliance of the technique, but couldn’t reward him with a good grade because it missed the essence of what he was tested for. It was a different style of work, a different style of jurisprudence.
Something-Else-First AI organizations work similarly. Instead of training each individual employee to do the same task, perhaps in a way similar to those of the past, perhaps with some new nuance, organizations capture past judgments and actions across a wide base of former employees and use these judgments - these secondary sources - to inform current actions. With enough data to train an algorithm, the actions might be completely automated. Most often there’s not enough to achieve satisfactory accuracy in the predictions, and organizations instead present guesses to current employees, who can provide feedback to improve performance in the future.
This ability to recycle past judgments and actions is very powerful. Outside enterprise applications, AI’s ability to fast forward our ability to stand on the shoulders of giants is shifting our direction as a species. Feedback loops like filtering algorithms on social media sites have the potential to keep us mired in an infantile past, with consequences that have been dangerous for democracy. We have to pay attention to that, as news and the exchange of information, all the way back to de Tocqueville, has always been key to democracy. Expanding self-reflexive awareness broadly across different domains of knowledge will undoubtedly change how disciplines evolve going forward. I remain hopeful, but believe we have some work to do to prepare the citizenship and workforce of the future.
*Image recognition algorithms do a great job showing why it’s dangerous for an AI company to bank its differentiation and strategy on an algorithmic capability as opposed to a unique ability to solve a business problem or amass a proprietary data set. Just two years ago, image recognition was a breakthrough capability just making its way to primetime commercial use. This June, Google released image recognition code for free via its Tensorflow API. That’s a very fast turnaround from capability to commodity, a transition of great interest to my former colleagues at Fast Forward Labs.
**See here for ethical implications of this backward-looking temporality.
The featured image comes from a twelfth-century manuscript by neo-platonist philosopher Bernard de Chartres. It illustrates this quotation:
“We are like dwarfs on the shoulders of giants, so that we can see more than they, and things at a greater distance, not by virtue of any sharpness of sight on our part, or any physical distinction, but because we are carried high and raised up by their giant size.”
It’s since circulated from Newton to Nietzsche, each indicating indebtedness to prior thinkers as inspiration for present insights and breakthroughs.
Hi, I just had a dream, literally, and woke up thinking about the implications. Maybe you, or one of your computer genius friends, can prevent such a dream from becoming reality. I dreamed that the average person could alter the content of any website or document simply by moving an icon over another icon. The only way to prevent such perversions of the original document was for an “Integrity Shield” to be invented. We already have problems with photoshop mischief. What if every printed word were that easily vulnerable, too? Sometimes my dreams never come true; but sometimes they are warnings or encouragements from God that reveal bits of the truth in the future.
LikeLike
Wow, vivid dream. This reminds me of new capabilities to generate speech, which makes it even harder to discern fake news from real news: https://www.theverge.com/2017/7/12/15957844/ai-fake-video-audio-speech-obama. The ease of changing content in your dream with the hover ability seems to be what makes it so scary. Blockchain may be the key to protecting the integrity of a document, at least at some point in time!
LikeLike
I am so glad someone is working on it. Our church had a subsidiary website, in order to inform people about a backpack project, giving hundreds of backpacks, filled with supplies to kids, that got hacked. It would be neat if such things were preventable.
LikeLike