There’s all this talk that robots will replace humans in the workplace, leaving us poor, redundant schmucks with nothing to do but embrace the glorious (yet terrifying) creative potential of opiates and ennui. (Let it be noted that bumdom was all the rage in the 19th century, leading to the surging ecstasies of Baudelaire, Rimbaud, and the crown priest of hermeticism (and my all-time favorite poet besides Sappho*), Stéphane Mallarmé**).
As I’ve argued in a previous post, I think that’s bollocks. But I also think it’s worth thinking about what cognitive, services-oriented jobs could and should look like in the next 20 years as technology advances. Note that I’m restricting my commentary to professional services work, as the manufacturing, agricultural, and transportation (truck and taxi driving) sectors entail a different type of work activity and are governed by different economic dynamics. They may indeed be quite threatened by emerging artificial intelligence (AI) technologies.
So, here we go.
I’m currently reading Yuval Noah Harari’s latest book, Homo Deus, and the following passage caught my attention:
“In fact, as time goes by it becomes easier and easier to replace humans with computer algorithms, not merely because the algorithms are getting smarter, but also because humans are professionalizing. Ancient hunter-gatherers mastered a very wide variety of skills in order to survive, which is why it would be immensely difficult to design a robotic hunter-gatherer. Such a robot would have to know how to prepare spear points from flint stones, find edible mushrooms in a forest, track down a mammoth and coordinate a charge with a dozen other hunters, and afterwards use medicinal herbs to bandage any wounds. However, over the last few thousand years we humans have been specializing. A taxi driver or a cardiologist specializes in a much narrower niche than a hunter-gatherer, which makes it easier to replace them with AI. As I have repeatedly stressed, AI is nowhere near human-like existence. But 99 per cent of human qualities and abilities are simply redundant for the performance of most modern jobs. For AI to squeeze humans out of the job market it needs only to outperform us in the specific abilities a particular profession demands.”
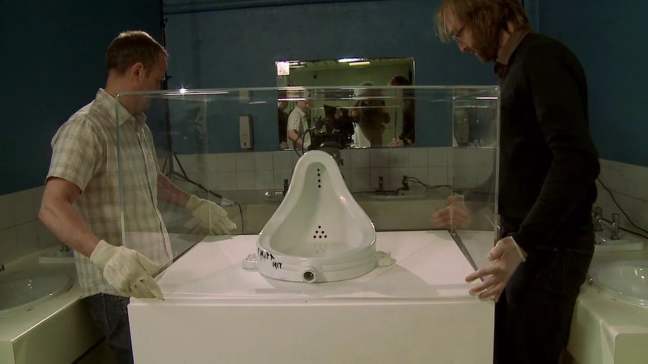
This is astute. I love how Harari debunks the false impression that the human race progresses over time. We tend to be amazed upon seeing the technical difficulty of ancient works of art at the Met or the Louvre, assuming History (big H intended) is a straightforward, linear march from primitivism towards perfection. While culture and technologies are passed down through language and traditions from generation to generation, shaping and changing how we interact with one another and with the physical world, how we interact as a collective and emerge into something way beyond our capacities to observe, this does not mean that the culture and civilization we inhabit today is morally superior to those that came before, or those few that still exist in the remote corners of the globe. Indeed, primitive hunter-gatherers, given the broad range of tasks they had to carry out to survive prior to Adam Smith’s division of labor across a collective, may have a skill set more immune to the “cognitive” smarts of new technologies than a highly educated, highly specialized service worker!
This reveals something about both the nature of AI and the nature of the division of labor in contemporary capitalism arising from industrialism. First, it helps us understand that intelligent systems are best viewed as idiot savants, not Renaissance Men. They are specialists, not generalists. As Tom Mitchell explains in the opening of his manifesto on machine learning:
“We say that a machine learns with respect to a particular task T, performance metric P, and type of experience E, if the system reliably improves its performance P at task T, following experience E. Depending on how we specify T, P, and E, the learning task might also be called by names such as data mining, autonomous discovery, database updating, programming by example, etc.”
Confusion about super-intelligent systems stems from the popular misunderstanding of the word “learn,” which is a term of art with a specific meaning in the machine learning community. The learning of machine learning, as Mitchell explains, does not mean perfecting a skill through repetition or synthesizing ideas to create something new. It means updating the slope of your function to better fit new data. In deep learning, these functions need not be simple, 2-D lines like we learn in middle school algebra: they can be incredibly complex curves that transverse thousands of dimensions (which we have a hard time visualizing, leading to tools like t-SNE that compress multi-dimensional math into the comfortable space-time parameters of human cognition).
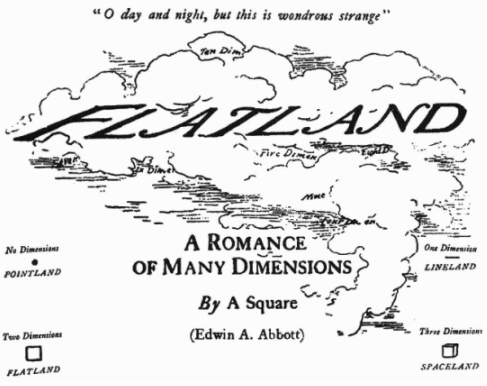
The AI research community is making baby steps in the dark trying to create systems with more general intelligence, i.e., systems that reliably perform more than one task. OpenAI Universe and DeepMind Lab are the most exciting attempts. At the Future Labs AI Summit this week, Facebook’s Yann LeCun discussed (largely failed) attempts to teach machines common sense. We tend to think that highly skilled tasks like diagnosing pneumonia from an X-ray or deeming a tax return in compliance with the IRS code require more smarts than intuiting that a Jenga tower is about to fall or perceiving that someone may be bluffing in a poker game. But these physical and emotional intuitions are, in fact, incredibly difficult to encode into mathematical models and functions. Our minds are probabilistic, plastic approximation machines, constantly rewiring themselves to help us navigate the physical world. This is damn hard to replicate with math, no matter how many parameters we stuff into a model! It may also explain why the greatest philosophers in history have always had room to revisit and question the givens of human experience****, infinitely more interesting and harder to describe than the specialized knowledge that populates academic journals.
Next, it is precisely this specialization that renders workers susceptible to being replaced by machines. I’m not versed enough in the history of economics to know how and when specialization arose, but it makes sense that there is a tight correlation between specialization, machine coordination, and scale, as R. David Dixon recently discussed in his excellent Medium article about machines and the division of labor. Some people are drawn to startups because they are the antithesis of specialization. You get to wear multiple hats, doubling, as I do in my role at Fast Forward Labs, as sales, marketing, branding, partnerships, and even consulting and services delivery. Guild work used to work this way, as in the nursery rhyme Rub-a-dub-dub: the butcher prepared meat from end to end, the baker made bread from end to end, and the candlestick maker made candles from end to end. As Dixon points out, tasks and the time it takes to do tasks become important once the steps in a given work process are broken apart, leading to theories of economic specialization as we see in Adam Smith, Henry Ford, and, in their modern manifestation, the cold, harsh governance of algorithms and KPIs. The corollary of scale is mechanism, templates, repetition, efficiency. And the educational system we’ve inherited from the late 19th century is tailored and tuned to farm out skilled, specialized automatons who fit nicely into the specific roles required by corporate machines like Google or Goldman Sachs.
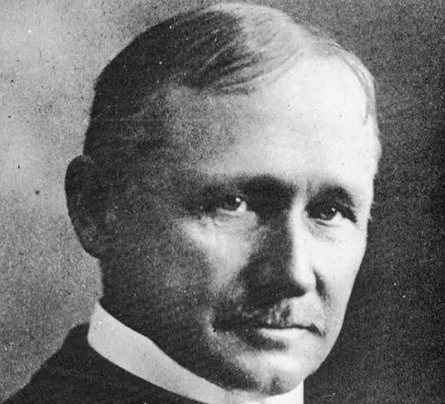
This leads to the core argument I’d like to put forth in this post: the right educational training and curriculum for the AI-enabled job market of the 21st century should create generalists, not specialists. Intelligent systems will get better and better at carrying out specific activities and specific tasks on our behalf. They’ll do them reliably. They won’t get sick. They won’t have fragile egos. They won’t want to stay home and eat ice cream after a breakup. They can and should take over this specialized work to drive efficiencies and scale. But, machines won’t be like startup employees any time soon. They won’t be able to reliably wear multiple hats, shifting behavior and style for different contexts and different needs. They won’t be creative problem solvers, dreamers, or creators of mission. We need to educate the next generation of workers to be more like startup employees. We need to bring back respect for the generalist. We need the honnête homme of the 17th century or Arnheim*** in Robert Musil’s Man Without Qualities. We need hunter-gatherers who may not do one thing fabulously, but have the resiliency to do a lot of things well enough to get by.
What types of skills should these AI-resistant generalists have and how can we teach them?
Flexibility and Adaptability
Andrew Ng is a pithy tweeter. He recently wrote: “The half-life of knowledge is decreasing. That’s why you need to keep learning your whole life, not only through college.”
This is sound. The apprenticeship model we’ve inherited from the guild days, where the father-figure professor passes down his wisdom to the student who becomes assistant professor then associate professor then tenured professor then stays there for the rest of his life only to repeat the cycle in the next generation, should probably just stop. Technologies are advancing quickly, which open opportunities to automate tasks that we used to do manually or do new things we couldn’t do before (like summarizing 10,000 customer reviews on Amazon in a second, as the system my colleagues at Fast Forward Labs built). Many people fear change and there are emotional hurdles to having to break out of habits and routine and learn something new. But honing the ability to recognize that new technologies are opening new markets and new opportunities will be seminal to succeeding in a world where things constantly change. This is not to extol disruption. That’s infantile. It’s to accept and embrace the need to constantly learn to stay relevant. That’s exciting and even meaningful. Most people wait until they retire to finally take the time to paint or learn a new hobby. What if work itself offered the opportunity to constantly expand and take on something new? That doesn’t mean that everyone will be up to the challenge of becoming a data scientist over night in some bootcamp. So the task universities and MOOCs have before them is to create curricula that will help laymen update their skills to stay relevant in the future economy.
Interdisciplinarity
From the late 17th to mid 18th centuries, intellectual giants like Leibniz, D’Alembert, and Diderot undertook the colossal task of curating and editing encyclopedias (the Greek etymology means “in the circle of knowledge”) to represent and organize all the world’s knowledge (Google and Wikipedia being the modern manifestations of the same goal). These Enlightenment powerhouses all assumed that the world was one, and that our various disciplines were simply different prisms that refracted a unified whole. The magic of the encyclopedia lay in the play of hyperlinks, where we could see the connections between things as we jumped from physics to architecture to Haitian voodoo, all different lenses we mere mortals required to view what God (for lack of a better name) would understand holistically and all at once.
Contemporary curricula focused on specialization force students to grow myopic blinders, viewing phenomena according to the methodologies and formalisms unique to a particular course of study. We then mistake these different ways of studying and asking questions for literally different things and objects in the world and in the process develop prejudices against other tastes, interests, and preferences.
There is a lot of value in doing the philosophical work to understand just what our methodologies and assumptions are, and how they shape how we view problems and ask and answer questions about the world. I think one of the best ways to help students develop sensitivities for methodologies is to have them study a single topic, like climate change, energy, truth, beauty, emergence, whatever it may be, from multiple disciplinary perspectives. So understanding how physics studies climate change; how politicians study climate change; how international relations study climate change; how authors have portrayed climate change and its impact on society in recent literature. Stanford’s Thinking Matters and the University of Chicago’s Social Thought programs approach big questions this way. I’ve heard Thinking Matters has not helped humanities enrollment at Stanford, but still find the approach commendable.
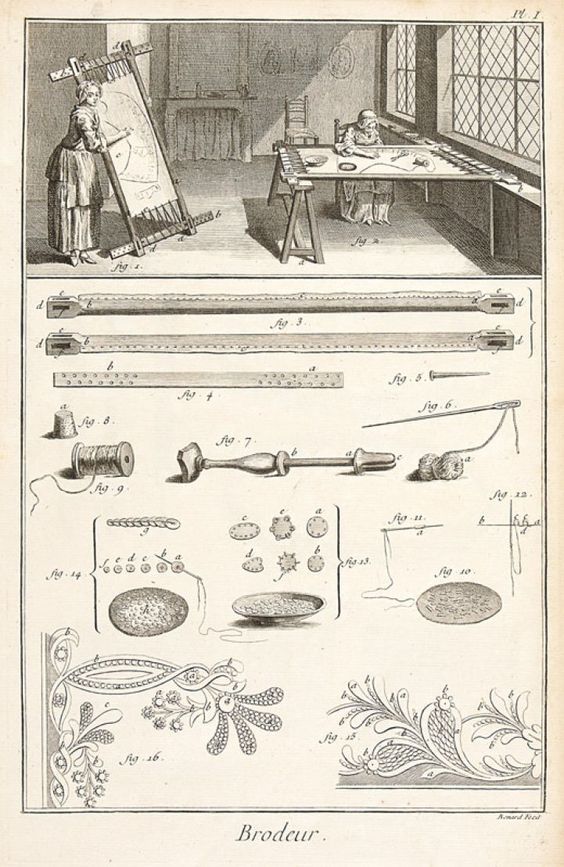
Model Thinking
Michael Lewis does a masterful job narrating the lifelong (though not always strong) partnership between Daniel Kahneman and Amos Tversky in The Undoing Project. Kahneman and Tversky spent their lives showing how we are horrible probabilistic thinkers. We struggle with uncertainty and have developed all sorts of narrative and heuristic mental techniques to make our world make more concrete sense. Unfortunately, we need to improve our statistical intuitions to succeed in the world of AI, which are probabilistic systems that output responses couched in statistical terms. While we can hide this complexity behind savvy design choices, really understanding how AI works and how it may impact our lives requires that we develop intuitions for how models, well, model the world. At least when I was a student 10 years ago, statistics was not required in high school or undergrad. We had to take geometry, algebra, and calculus, not stats. It seems to make sense to make basic statistics a mandatory requirement for contemporary curricula.
Synthetic and Analogical Reasoning
There are a lot of TED Talks about brains and creativity. People love to hear about the science of making up new things. Many interesting breakthroughs in the history of philosophy or physics came from combining together two strands of thought that were formerly separate: the French psychoanalyst Jacques Lacan, whose unintelligibility is besides the point, cleverly combined linguistic theory from Ferdinand Saussure with psychoanalytic theory from Sigmund Freud to make his special brand of analysis; the Dutch physicist Erik Verlinde cleverly combined Newton and Maxwell’s equations with information theory to come to the stunning conclusion that gravity emerges from entropy (which is debated, but super interesting).
As we saw above, AI systems aren’t analogical or synthetic reasoners. In law, for example, they excel at classification tasks to identify if a piece of evidence is relevant for a given matter, but they fail at executing other types of reasoning tasks like identifying that the facts of a particular case are similar to the facts of another to merit a comparison using precedent. Technology cases help illustrate this. Data privacy law, for example, frequently thinks about our right to privacy in the virtual world through reference back to Katz v. United States, a 1967 case featuring a man making illegal gambling bets from a phone booth. Topic modeling algorithms would struggle to recognize that words connoting phones and bets had a relationship to words connoting tracking sensors on the bottom of trucks (as in United States v. Jones). But lawyers and judges use Katz as precedent to think through this brave new world, showing how we can see similarities between radically different particulars from a particular level of abstraction.
Does this mean that, like stats, everyone should take a course on the basics of legal reasoning to make sure they’re relevant in the AI-enabled world? That doesn’t feel right. I think requiring coursework in the arts and humanities could do the trick.
Framing Qualitative Ideas as Quantitative Problems
A final skill that seems paramount for the AI-enabled economy is the ability to translate an idea into something that can be measured. Not everyone needs to be able to this, but there will be good jobs-and more and more jobs-for the people who can.
This is the data science equivalent of being able to go from strategy to tactical execution. Perhaps the hardest thing in data science, in particular as tooling becomes more ubiquitous and commoditized, is to figure out what problems are worth solving and what products are worth building. This requires working closely with non-technical business leaders who set strategy and have visions about where they’d like to go. But it takes a lot of work to break down a big idea into a set of small steps that can be represented as a quantitative problem, i.e., translated into some sort of technology or product. This is also synthetic and interdisciplinary thinking. It requires the flexibility to speak human and speak machine, to prioritize projects and have a sense for how long it will take to build a system that does what need it to do, to render the messy real-world tractable for computation. Machines won’t be automating this kind of work anytime soon, so it’s a skill set worth building. The best way to teach this is through case studies. I’d advocate for co-op training programs alongside theoretical studies, as Waterloo provides for its computer science students.
Conclusion
While our culture idealizes and extols polymaths like Da Vinci or Galileo, it also undervalues generalists who seem to lack the discipline and rigor to focus on doing something well. Our academic institutions prize novelty and specialization, pushing us to focus on earning the new leaf at the edge of a vast tree wizened with rings of experience. We need to change this mindset to cultivate a workforce that can successfully collaborate with intelligent machines. The risk is a world without work; the reward is a vibrant and curious new humanity.
The featured image is from Émile, Jean-Jacques Rousseau’s treatise on education. Rousseau also felt educational institutions needed to be updated to better match the theories of man and freedom developed during the Enlightenment. Or so I thought! Upon reading this, one of my favorite professors (and people), Keith Baker, kindly insisted that “Rousseau’s goal in Emile was not to show how educational institutions could be improved (which he didn’t think would be possible without a total reform of the social order) but how the education of an individual could provide an alternative (and a means for an individual to live free in a corrupt society).” Keith knows his stuff, and recalling that Rousseau is a misanthropic humanist makes things all the more interesting.
*Sappho may be the sexiest poet of all time. An ancient lyric poet from Lesbos, she left fragments that pulse with desire and eroticism. Randomly opening a collection, for example, I came across this:
Afraid of losing you
I ran fluttering/like a little girl/after her mother
**I’m stretching the truth here for rhetorical effect. Mallarmé actually made a living as an English teacher, although he was apparently horrible at both teaching and speaking English. Like Knausgaard in Book 2 of My Struggle, Mallarmé frequently writes poems about how hard it is for him to find a block of silence while his kids are screaming and needing attention. Bourgeois family life sublimated into the ecstasy of hermeticism. Another fun fact is that the French Symbolists loved Edgar Allen Poe, but in France they drop the Allen and just call him Edgar Poe.
***Musil modeled Arnheim after his nemesis Walther Rathenau, the German Foreign Minister during the Weimar Republic. Rathenau was a Jew, but identified mostly as a German. He wrote some very mystical works on the soul that aren’t worth reading unless you’d like to understand the philosophical and cocktail party ethos of the Habsburg Empire.
****I’m a devout listener of the Partially Examined Life podcast, where they recently discussed Wilfrid Sellars’s Empiricism and the Philosophy of Mind. Sellars critiques what he calls “the myth of the given” and has amazing thoughts on what it means to tell the truth.